Cross Currents Fall 2023 Alumni Feature Story: Jeremy Cohen ’12
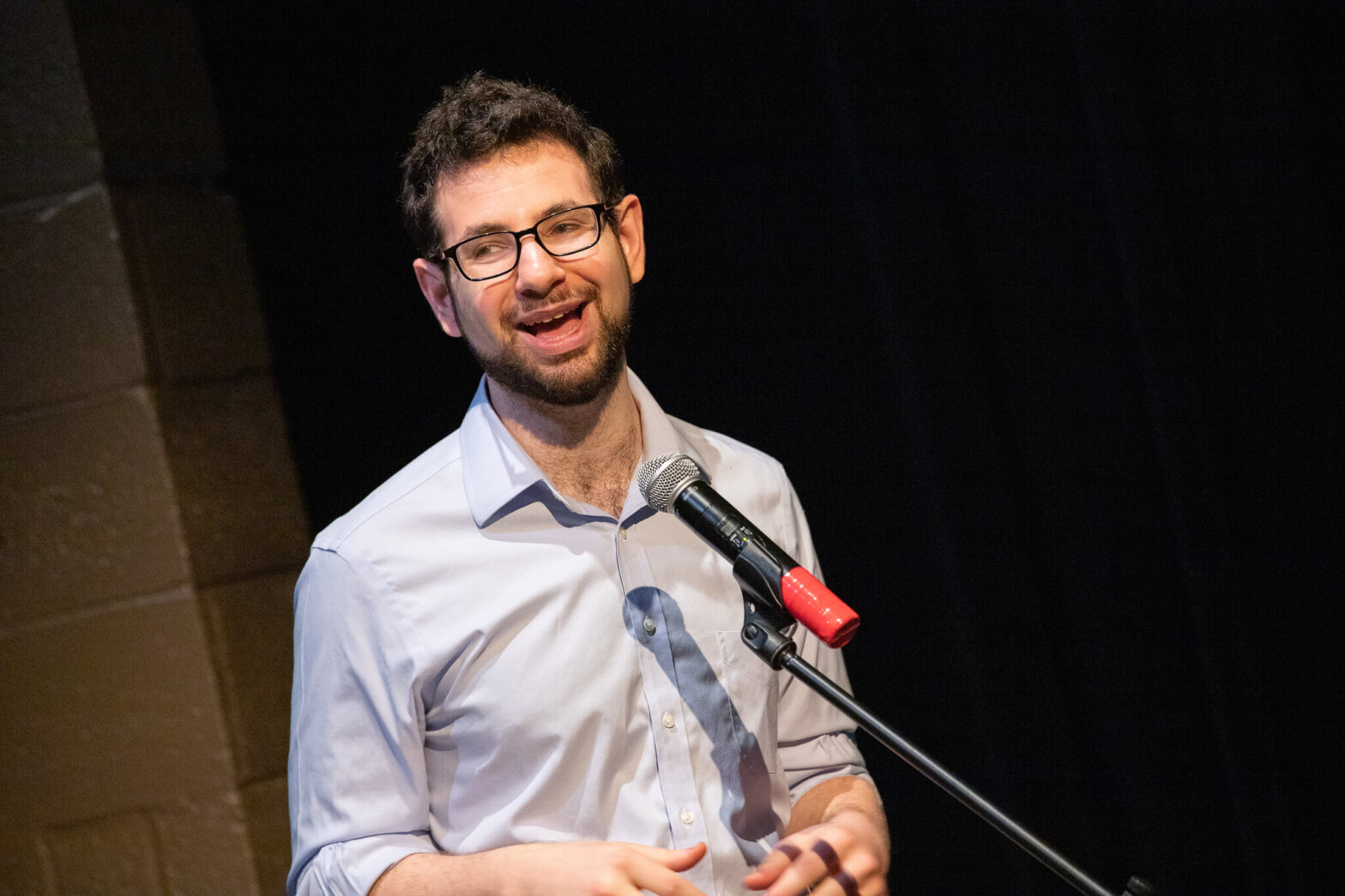
“Machine learning is in a weird state where we’ve figured out how to do stuff that works, but we don’t really understand why,” says Jeremy Cohen, Class of 2012. Jeremy is currently in his sixth year of a Ph.D. program at Carnegie Mellon University, studying machine learning.
While he was always interested in computer science as a student at Park, Jeremy’s interest in machine learning really ignited when he got to college at Princeton University and took a class on the subject.
Jeremy explains that machine learning studies how to get computers to do important things that can be helpful to people in their daily lives. “In general, you have to program computers by telling them explicitly what to do. This is easy for some tasks — adding large numbers together, for example — because you can articulate a procedure to follow. But for other tasks, like recognizing objects, it’s not possible to write down rules for a computer.”
“Humans learn by exposure, but we can’t turn that kind of learning into a systematic procedure that computers can follow. When you look at a picture, and you see it’s a dog, that happens automatically in the early stages of your brain processing. But to a computer, an image is just a series of pixels. To go from seeing pixels to knowing it’s an object is a different story altogether. Nobody has ever figured out a way to come up with a procedure for doing that that can be explicitly taught to a computer. As humans, we do it intuitively, but we don’t know how to describe what we’re doing. Many things fall in the category of we can do it, but we don’t know how to articulate what we do. The question of machine learning is always how to get computers to do these things.” In self-driving cars, for example, a car needs to know how to recognize a person from a car, or is that a red light or a green light?
The pivotal class Jeremy took in college explored an approach that gives computers a dataset of examples — many millions of examples — and has the computer learn what to do through exposure. “I took this class in undergrad and I thought it was the coolest thing in the world.”
And he still finds it fascinating. After working at Spotify for a few years as a software engineer, Jeremy decided he wanted to venture into the world of research. He wasn’t always sure he wanted to pursue graduate school, and while he explored tech internships in college, it was actually David Weiss ’03, another Millhauser Fellow working in machine learning, who gave him the right type of advice that helped him decide what he wanted to do. Jeremy remembered hearing him speak at one of the Millhauser assemblies at Park, so he reached out to him for career advice, and that email exchange motivated Jeremy to apply for Ph.D. programs.
Into the world of machine learning he went at Carnegie Mellon University. He spends his days researching, but his research is very unlike that of most of his peers. “Most researchers have a particular task that they’re trying to get AI (artificial intelligence) to do well on, and they’ll spend their days fiddling with stuff until AI starts to work on that task. I’m looking at tasks that were solved 10-15 years ago, but we still don’t understand why our solution works. I’m trying to understand why this thing works.” It’s unusual, he says. There aren’t many people who do things this way, but Jeremy spends his days carefully running computer experiments, seeing what happens, and checking that against math.
“My collaborators and I try to have the math explain what we see coming out of the computer. Our goal is to make the theory match the experiment — to come up with math that is predictive of what we observe.”
“I think AI and machine learning are going to be key technological advances of the coming decades. So it’s cool to be able to work on putting together theoretical foundations. It’s analogous to working on the computers in the 60s or 70s, or steam engines whenever those were coming onto the scene. Nobody really knows how the future’s going to play out with AI. What we can tell is obviously there are going to be good things about it and bad things. I hope that the good things will outweigh the bad.”
On the good side, AI will help people accomplish much more. “I’ve used AI myself in my own work, and it’s pretty amazing. If there’s a coding task that I want done, but don’t want to do it because it would require a coding language I don’t know, I can ask the AI system to write the code. So AI can be a huge productivity boost for me in my role.”
“I feel sorry for all of the teachers who have to cope with this because nobody knows what’s going to happen to school work. This is the first time that a computer can do real school work. Today it’s English essays and tomorrow it’s going to be physics questions. No one knows what’s going to happen with this.”
As a Park student who benefitted from experiencing the Millhauser Fellows program, to becoming a Fellow himself, he’s ready to help. “It was fun to be back, great to see a lot of my old teachers, and catch up with them. I hope that maybe in 5 or 10 years, I’ll help inspire someone else with career advice, just as I was.”
Find the full Fall 2023 issue of Cross Currents here. Learn more about the Millhauser Fellowship program here.
Back to The Latest